What Impact Does The Digital Revolution Have On Work And Inequality?
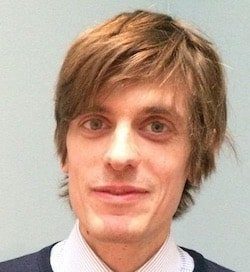
Michael Osborne
Okay Michael, thank you very
much for joining us today to have a discussion about innovation,
technological changes and what it means for the future of work. Together
with your colleague Carl Benedikt Frey, you’ve published several studies that have made a big impact. First
of all, can I ask you, when you write about the changing nature of
innovation, what in particular do you mean? What is changing?
Okay, so the developments about which
I’m excited have emerged from my own field, which is that of machine
learning, which many of your listeners might know better by the name of
artificial intelligence. Our idea is that developments in the field of
machine learning, accompanied by those in mobile robotics, are really
going to have quite a transformational impact upon human employment. In
particular, we point to the introduction of algorithms that are able to
perform sophisticated subtle decision-making in a way that was
previously the reserve of humans, that are able to navigate the streets,
that are able to make recommendations of products to customers.
All of these things are very likely to
substitute for human workers in the fairly near future. So, our study
set out to put some numbers to this phenomenon. In particular, we used a
data set from the US, in which we had 702 different occupational
classes and for each of those we had a list of characteristics of the
job.
So, this organisation in the US had gone
about determining their requirements for different types of skills
within each of its different occupations. These included things like
persuasion, manual dexterity, originality – all characteristics that we
thought might be predictive of a job’s susceptibility to automation.
So, on the basis of that data, we used,
in fact, a machine-learning algorithm and the characteristics of jobs
about which we were most confident were not going to be automated in the
next 20 years, or that, in fact, we had already seen some evidence of
being automated, to try and identify the characteristics of jobs that
rendered them susceptible to automation and thereby to predict for all
jobs a probability of automation. In particular, we arrived at this
headline figure that 47% of current US employment might be at high risk
of automation over the next 20 years.
Okay and if you want to
categorise, in the international literature, there seems to be a
tendency that the jobs that are less likely or the least likely to be
substituted are ones based on creativity, even though there was a
discussion about it, but also the ones, in particular, based on social
capital and human interaction. Is this something you would also agree
with?
We agree entirely with that
characterisation. In our study we highlighted three bottlenecks to
computerisation, as we call them. They were exactly creativity, social
intelligence and perception or manipulation. So, what unites those three
bottlenecks is the deep reservoirs of tacit knowledge that humans have about the task they’re performing.
To give an example, consider the
generation of a hit song, a quintessentially creative task. It’s very
easy to get an algorithm to churn out an endless stream of songs, but
it’s very difficult to teach the algorithm the difference between a hit
song and not such a good song.
Because, in doing so, as a human we draw
upon this enormously sophisticated conception of our social environment
and our cultural context, in a way that’s very difficult to explicitly
state in code. So, it’s exactly those three characteristics of jobs that
we think are most difficult to automate.
The German Labour Minister has
recently commissioned a study that took a slightly different view of the
automation potential. They have basically three main points of
criticism of your methodology. The first one would be what you seem to
be describing is more an impact of technology on ‘activities’, rather
than ‘jobs’ themselves.
The same jobs might not even
perform the same activity, so there is a difference between the activity
level and the actual job level. The second one is that you estimate the
potential that you rely on that’s too much based on expert opinion,
which tends to overestimate the potential for substitution and
rationalisation.
The third aspect was that even
though there might be this kind of technological potential, there are
also social, legal as well as ethical barriers to implementation, which
would mean that the full potential will not be achieved. So, the figures
they came up with is 9% in the US and 12% of German jobs might be at
risk of automation. What is your response to that kind of approach?
Well, my response to the second of those
criticisms first, because it’s the only one with which I really
disagree. They highlight our use of expert opinion as a basis for our
study, which is true, but I think more needs to be said about that.
The first point is that we weren’t slavishly following the advice of the experts upon which we drew. The
way our algorithm worked, which is itself a quite sophisticated piece
of machine learning, is that the advice of the experts, the labels they
provided to 70 different occupations, as being either automatable or not
over the next 20 years, were treated as noisy or untrustworthy
estimates of what will actually happen in reality.
So, our algorithm is actually quite
tolerant to mis-labelled examples. To give one kind of example: we
included waiters and waitresses as an example of an occupation that was
non-automatable and I guess the thinking there was that the kind of
small talk that a waiter or waitress makes in the process of performing
their job is not something that’s readily automated. That it’s very
difficult to teach an algorithm all the social nuances that a waiter or
waitress might draw upon.
Nonetheless, our algorithm had come back
and told us that the probability of computerisation for waiters and
waitresses was 94%, which struck us as quite high. But interestingly, in
the time since we’ve published the studies, so since 2013, we have
actually seen restaurants, particularly in the US, automating some of
the jobs that waiters and waitresses might have traditionally been asked
to perform, in placing tablets on tables in restaurants.
So, these tablets were able to take
customers’ orders, they were able to recommend products to them, in
fact, they were able to substantially increase dessert sales in
particular, by as much as 30%, by providing customers with these
enticing looking images on the tablet.
So, the point I was trying to make is
that the algorithm we use wasn’t completely subject to the biases that
the experts we used provided us. It was able to correct, as I say, for
some mistaken labels. In particular, we performed some sensitivity
analysis in which we sub-divided the training set that we used, so, in
particular, we used a sub-set of 35 of the 70 occupations that we had
labelled to try and predict the other 35.
We did this over many iterations of
splitting that set into two. Yet, regardless of the split that we made,
we found that we were able to very accurately predict that held out
half, given the half that we’d retained for the algorithm. So, what this
tells us is that, yes, it’s possible that we’re wrong entirely about
what is automatable, but at least we’re consistently wrong, that there
doesn’t seem to be a whole lot of inconsistency in this set that was
provided.
Okay and would you say it’s basically that for some of the jobs you were actually on the cautious side?
I think that’s true, yes. So, for
waiters and waitresses as one characteristic example, we’d
over-estimated the influence of social intelligence, but what the
algorithm picked up is that the low creativity score, or originality
score for waiters and waitresses does indeed render them quite
susceptible to automation.
Okay. What do you make of the
argument that the likely impact is not necessarily substitution only,
but it’s basically a changing face of the job, so that the job
description itself will change and the human activity will basically be
more augmenting technological capacity, rather than being in direct
competition. Is this also something that you would subscribe to?
I’m very sympathetic to that point of
view; I think that is indeed how automation takes root in the economy.
So, historically, of course, we saw people like the typing pool of the
1950s replaced by word processing software, despite the fact that it’s
not that the word processing software was able to do everything that the
typing pool was able to do.
The job was simplified to the point that
it was able to be automated. So, the job of what that typing pool
contained was essentially eliminated despite all its characteristics not
being automatable. But, more broadly, there was a study recently from
Australia, which tried to put some numbers to this phenomenon by which
the characteristics of a job might actually change over time and, in
particular, how they might change in relation to automatability.
This study will be coming out of the
office of the chief economist in Australia, it had found that there have
indeed been quite substantial changes within occupations, as pertaining
to their susceptibility to automation, even over the last ten years.
So, I think that is a very substantial kind of effect.
I’m not sure whether the real world
consequences will mean that occupations become either more or less
automatable, I guess my first point was that, in fact, it’s possible to
simplify tasks, simplify jobs to the point that they are automatable,
just as jobs are able to be rendered more complex to the point that
they’re not automated and which of these will actually play out in the
real world is still up for debate.
So we’re basically talking
about, when it comes to the impact of technology on jobs, three levels.
The first level would be what kinds of jobs could be replaced by
technology, the second one would be what kinds of jobs will change,
maybe even fundamentally, because of the impact of technology and the
third one would be job creation, what kinds of jobs will be newly
created as a result of technology. What would you say to the third
point?
So, again, a very important point we
quite explicitly acknowledged in our original study is that it did not
consider the prospect for new job growth. However, in subsequent work,
my co-author Carl Benedikt Frey has indeed looked at what new jobs have
emerged over the last decade within the US and finds, quite alarmingly,
that the new industries that have been created now only contain about
half of one percent of current US employment.
So, these new industries that have
emerged haven’t necessarily generated a whole host of new employment and
perhaps the most emblematic example is found in the tech sector, where a
company like WhatsApp, for example, was bought out for 19 billion US,
at a point at which it had only 55 employees, which compares quite
unfavourably against a business of, kind of, similar valuation, like the
fashion retailer GAP, which has about 137,000 employees.
So, yes, there are new jobs being
created, but it’s not clear that there are as many of those new jobs as
we might like and it’s also not clear that the jobs that are being
created might be suitable for the people who are put out of work due to
trends in technology.
So, if we look at the list of fastest
growing occupations since the year 2000, they include jobs like data
scientists, IOS developers, Android developers. I don’t really think
that there are going to be all that many people put out of work by, for
example, self-driving vehicles, people like truck drivers, who are able
to move very naturally into these quite high-skilled occupations.
So we might also see, basically, a polarisation of the labour market? I mean, when I discussed my own work on the impact of technology on work
on BBC Newsnight at the end of last year, Evan Davis, the presenter
said, “Well, we hadn’t had so many nail bars around previously,” so you
might say that there is a polarisation into high end, high skilled jobs,
but also very low skilled personal service sector jobs?
I think that’s absolutely right and, in
fact, one bit of analysis we did in our original study was to try and
plot the probability of computerisation against two different measures
of skill. So, in particular, we considered how the probability of
computerisation might relate to the fraction of workers in any
occupation who have at least a bachelor’s degree and also the average
median wage in each of these occupations.
What we saw, very clearly, is that the
probability of computerisation was negatively correlated with these
measures of skill, so simply put, the more skilled you are, the less
susceptible you are to computerisation, which does indeed suggest only a
continuation of this polarisation in the job market.
And a trend that we’ve seen even
before that, you know the sort of low skill, personalised service
sector jobs; they’re also very hard to replace with technology, aren’t
they?
That’s right, but they’re not
necessarily going to be very highly paid, because as the result of them
not requiring much skill, there’s going to be a whole host of people who
are willing to take on these jobs and this, as I suggested, may only
lead to an exasperation of the inequality we’ve seen develop over the
last couple of decades.
Yes, exactly, what it basically
means is that the middle skilled jobs are going to be hollowed out,
they’re moving towards both ends, to the very top and the very bottom.
I think that’s correct.
You’ve just mentioned, actually,
the next aspect I want to talk to you about, it’s basically inequality.
The main point of the paper that I wrote on the issue, at the end of
last year, is that we are already back to inequality levels that show
certain macroeconomic dysfunctionalities. I mean, you just have to go
into Thomas Piketty’s work to look at the fundamentals of this.
But, there is a likelihood with
the unfolding impact of technology that these inequalities will get
worse, at least in the short to medium term and that we’re going to see
an exacerbation of inequality. Would you also see it that way?
I would indeed and, in fact, there is an excellent book that’s just come out from Oxford economist, Tony Atkinson,
which puts even more weight behind this hypothesis. I think we need to,
however, not lose sight of the fact that there will be enormous wealth
generated by technological change.
So, it’s clear that in the development
of digital goods that have next to zero marginal cost that innovation
has enormous potential of social good for all, at least when we consider
people as consumers. But the risk, of course, is that due to these
risks to people as workers, there will be developing inequality. I think
it’s not necessarily a problem of wealth generation, it’s a problem of
distribution.
Fundamentally, yes, it makes the
distribution question that we already have even more pressing, in my
view. Coming to the policy responses, I agree with everything you’ve
said and, in terms of policy response, how should policy-makers react to
this or try to prepare for this?
I personally see three areas
that need addressing. The first one is the distribution of the remaining
work, so there is an argument in the sense that we are back to where
John Maynard Keynes was several decades ago, about the need to work
longer hours or fewer hours.
So, there might be a question
about distributing the remaining work more evenly amongst the population
and allowing for more free time, which is basically something that, for
instance, German labour market studies tell you is what a lot of people
want.
The second would be, especially
if you want to prepare for the kind of augmentation role of humans
through technology, that the educational profiles need to change. So,
educational policy needs to prepare people much more in terms of
adaptability. That, to me, means that they need to be better at creative
and analytical skills rather than memorising facts, which a lot of the
educational systems still seems to rely on.
The third one is really dealing
with the social as well as economic consequences of this inequality
issue. Is this something that you would broadly see as the categories
along which we would have to think and what would you add?
Well, I think you’re right, but I think
this problem is an enormously challenging one and I’m not sure there is a
simple silver bullet solution. Picking up on some of the suggestions in
particular, I think you’re right that education needs to change in
response to the accelerating rate of technological change, but how, in
particular, it should change, I think is a much thornier issue.
So, you’ve highlighted that, perhaps,
our schooling system should emphasise more creativity and put less
emphasis upon memorising facts, but there is a question, first, about
how you might do that and exactly what kind of creativity should be
instilled.
If we take the example of one of the
occupations that’s emerged that I mentioned earlier, data scientists, I
think actually, to be quite creative as a data scientist, you need to
have, at your fingertips, imprinted upon your brain, a large mass of
mathematical and statistical facts.
I’m not really clear that you can be
creative in that occupation without, at some point, having learnt quite a
large amount of knowledge and how that is best to be done, I’m not
entirely sure and if indeed that’s the right type of creativity for 20
years hence, I’m not entirely sure. I think it’s very difficult to
foresee exactly what skills, in particular, the workforce of 20 years
hence will need.
We’re not at a detailed level
here yet. At the beginning, these are just to set out, really, the broad
compartments, in terms of policy thinking on this. Of course, it’s a
very broad brush, but you have to categorise first before you can
actually drill down into the details.
I mean, in the distributional
question, we have this debate, actually originated from Silicon Valley,
about the basic income and its impact on how it can prop up domestic
demand in a situation where we have inequality that is going to run away
even further. It’s just basically about categorising the policy areas
we need to address and then look for individual solutions within.
And fair enough, obviously I endorse
that particular effort to categorise possible responses. I’m just
concerned that even within these categories, it’s not clear to me that
there is something that can actively solve this problem, or that can
solve the problem while being, kind of, politically acceptable.
I mean, maybe my pessimism here is borne
out of, I mean, I’m an engineer, at the end of the day. I’m not an
economist, so the extent to which people are pointing to me as being
somehow a source for these policy responses is, to me, already a sign of
some sort of desperation.
Okay. But, if you take this away
for a moment, your backdrop as an engineer, if you were a policy maker
and there were no restrictions to what you could do, what would –
against the backdrop of the current knowledge of the subject – your
response be?
So, the distributional question that you
highlight, I think, is the most important one. With the best
educational system in the world, I don’t think we’ll necessarily be able
to upskill everyone to the point that they’re able to fill these new
jobs that are created. So, then it really does become a question of
ensuring that the wealth that is generated is shared equally amongst all
members of society.
How that’s to be done, I’m not entirely
sure. I think the universal basic income is one kind of exciting
possibility. But my background doesn’t leave me best qualified to assess
the relative merits of these different proposals.
Okay, well Michael, thank you
very much for joining us today on this highly relevant topic and I’m
sure it’s not going to go away any time soon.
Well, I hope not, it’s an important one
and I hope there will continue to be a lot of discussion around it.
Thank you very much for having me.
No comments:
Post a Comment